The sophistication of data over the past two decades from “-omic” fields, digital, and electronic health sources has carved out an extraordinary new landscape for unraveling the molecular threads of cancer as a promising platform for more effective diagnostic and therapeutic strategies.
Deeply engaged in that enterprise is Pei Wang, PhD, Professor of Genetics and Genomic Sciences at the Icahn School of Medicine at Mount Sinai. Over the past eight years, she has served as lead investigator of the Proteogenomic Data Analysis Center (PGDAC) at Mount Sinai. The PGDAC is an integral part of the Clinical Proteomic Tumor Analysis Consortium (CPTAC), a National Cancer Institute (NCI) program for understanding the molecular basis of cancer through the application of proteogenomics, or large-scale proteome and genome analysis.
Dr. Wang and her team have already provided valuable insights into colon, breast, ovarian, and a broad range of other cancers. She has made contributions to more than 15 major studies published in Cell or Cancer Cell over the past eight years, with some findings now being investigated in clinical trials.
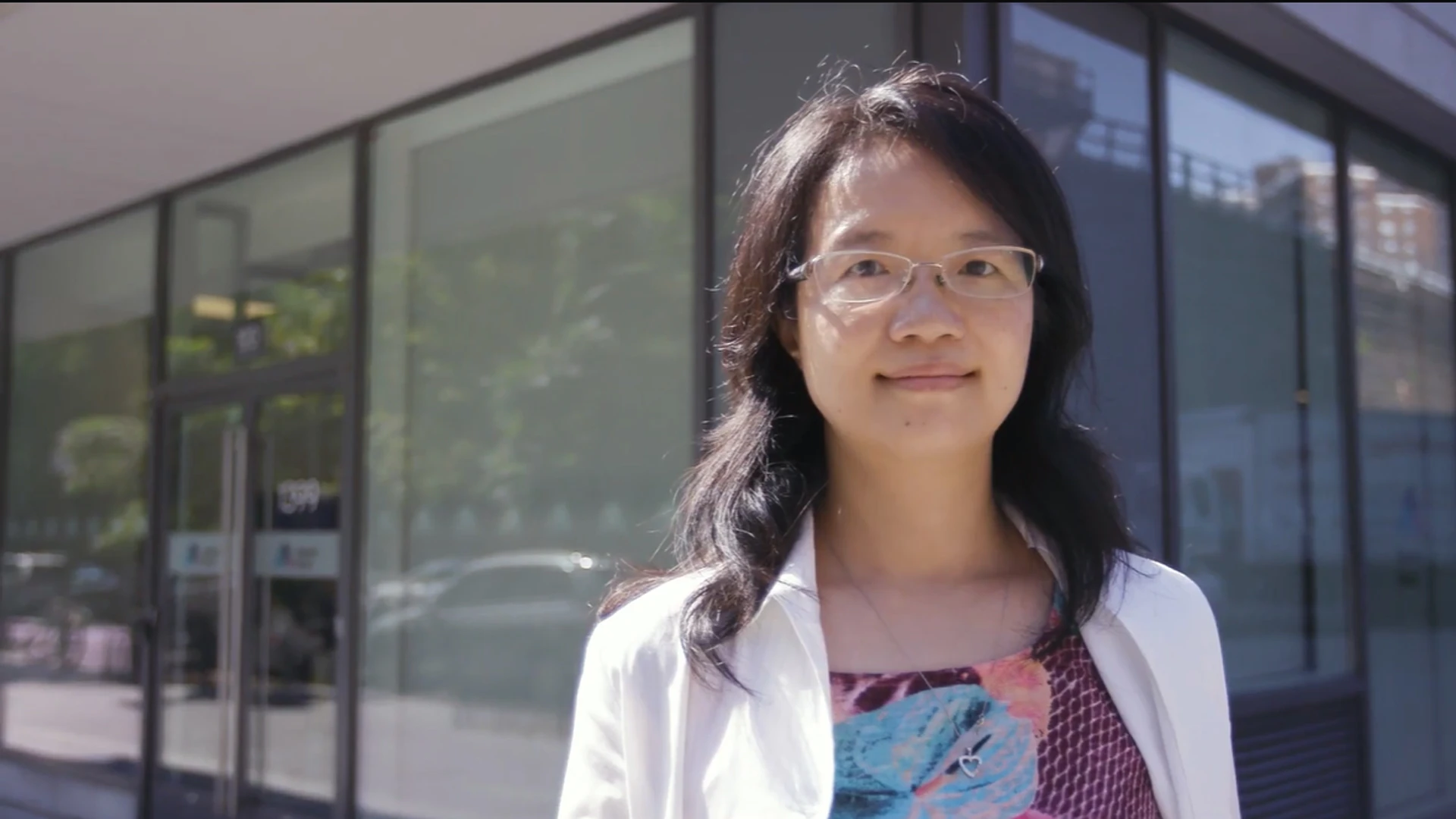
Pei Wang, PhD, is lead investigator of the Proteogenomic Data Analysis Center (PGDAC) at Mount Sinai, which is part of the Clinical Proteomic Tumor Analysis Consortium (CPTAC)—a National Cancer Institute (NCI) program for understanding the molecular basis of cancer.
“We want to understand the functional consequences of any mutation within a particular tumor cell for a patient, and proteomics data provides a novel window for that task beyond what’s been available to us in the past,” says Dr. Wang, who joined Mount Sinai as faculty in 2013. “From that knowledge, we can derive precise guidance on how to treat the patient—a sort of personalized medicine approach—as well as predict the outcome of that treatment based on our analysis of the multi-omics data of the tumor.”
Dr. Wang’s core areas of interest fit into two distinct categories: immune call surveillance/diverse immune evasion responses, and machine learning and deep learning techniques. The following is an overview of the vast work she does as part of CPTAC and as a computational scientist.
Immune Cell Surveillance and Diverse Immune Evasion Responses
Proteogenomic data can answer questions about kinase activities in different immune tumor subtypes. Understanding those activities could open doors to therapeutic targeting by small molecule drugs, and laying out what multi-omics profiles are predictive of poor or favorable responses to chemotherapy or immunotherapy.
With roughly no more than 20 percent of patients showing durable responses to a new generation of immunotherapies, there is a deep need for a better treatment landscape. Dr. Wang and her team recently performed a large-scale pan-cancer proteogenomic study, characterizing the immune composition of more than 1,000 tumors across 10 different cancer types using CPTAC’s unique multi-omics data set.
CPTAC, launched in 2006, encompasses PGDACs—of which Mount Sinai is one of four in the country—Proteome Characterization Centers, and Proteogenomic Translational Research Centers.
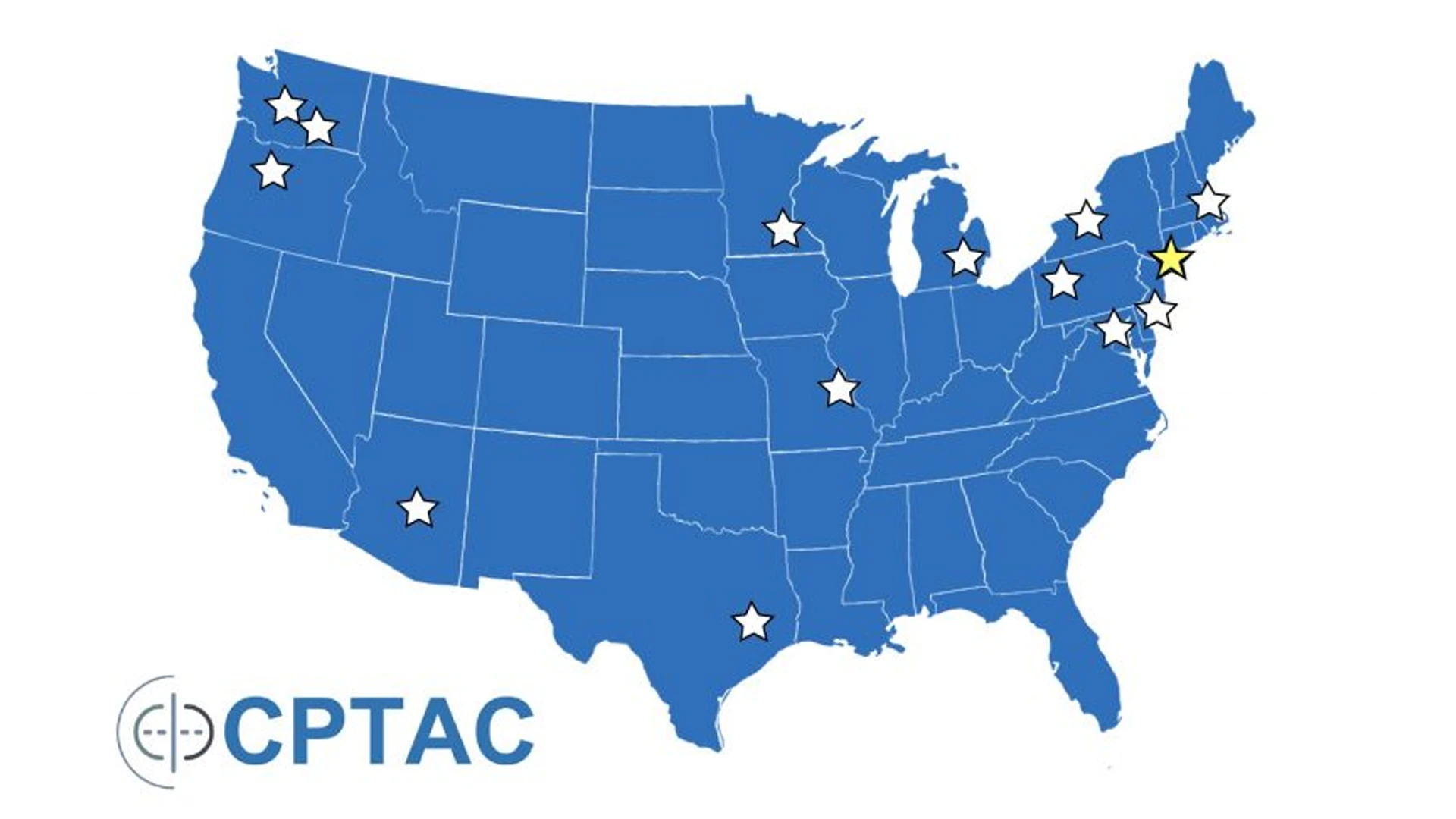
CPTAC comprises a network of Proteome Characterization Centers (PCCs), Proteogenomic Data Analysis Centers (PGDACs), and Proteogenomic Translational Research Centers (PTRCs)—a total of 14 centers nationwide. The Mount Sinai PGDAC conducts integration, visualization, and analyses using different data types (genomics, transcriptomics, and proteomics, imaging, and clinical data) to improve the understanding of genome-proteome relationship and the interplay/regulation of signaling pathways involved in cancer.
The large-scale study yielded an important discovery: despite the many differences across those 10 cancers, the multi-omics analysis revealed seven distinct immune subtypes based on integrative learning of cell type compositions and pathway activities. The team then categorized unique genomic, epigenetic, transcriptomic, and proteomic changes associated with each subtype.
“Our findings indicated a common molecular fingerprint of cancer patient immune response,” notes Dr. Wang, senior author of the study published in Cell in February 2024. The team further leveraged deep phosphoproteomic data to study kinase activity in different immune subtypes, and found known and potentially novel subtype-specific therapeutic targets.
“We believe this work appreciably advances our understanding of diverse immune activation and evasion strategies employed by tumors,” says Dr. Wang. “Just as importantly, it could lead to the development of novel immunotherapies while enhancing precision targeting with existing agents.”
Machine Learning and Deep Learning Techniques
New troves of multi-omics data requires new, advanced methods for analysis, and Dr. Wang’s team is constantly developing advanced machine learning methods and high-dimension network models to meet the challenge.
In particular, the researchers have been examining system-level behavior of DNA, RNA, and proteins to characterize their interactions, and, in turn, construct predictive models.
A recent significant study focused on chemo-refractory high-grade serous ovarian cancers (HGSOCs), which was done as part of CPTAC and published in Cell in 2023.
For patients with HGSOC, between 10 and 20 percent have treatment-refractory disease at diagnosis, fail to respond to initial therapy, and face a dismal prognosis. Moreover, there is currently no way to distinguish refractory from sensitive HGSOCs prior to therapy. Consequently, patients with refractory disease experience the toxicity of platinum-based chemotherapy without any benefit.
Dr. Wang and her colleagues used cutting-edge machine learning methodologies to map the proteogenomic landscape of 242 (refractory and sensitive) HGSOCs and, from that field, identify with high specificity a signature of 64 proteins that predicts poor response to first-line platinum-based therapy. More specifically, they pinpointed five subtypes of HGSOCs based on pathway protein expression, potentially reflecting different mechanisms of refractoriness while implicating subtype-specific treatment approaches that include immune therapies, TGF-β inhibitors, and metabolic inhibitors.
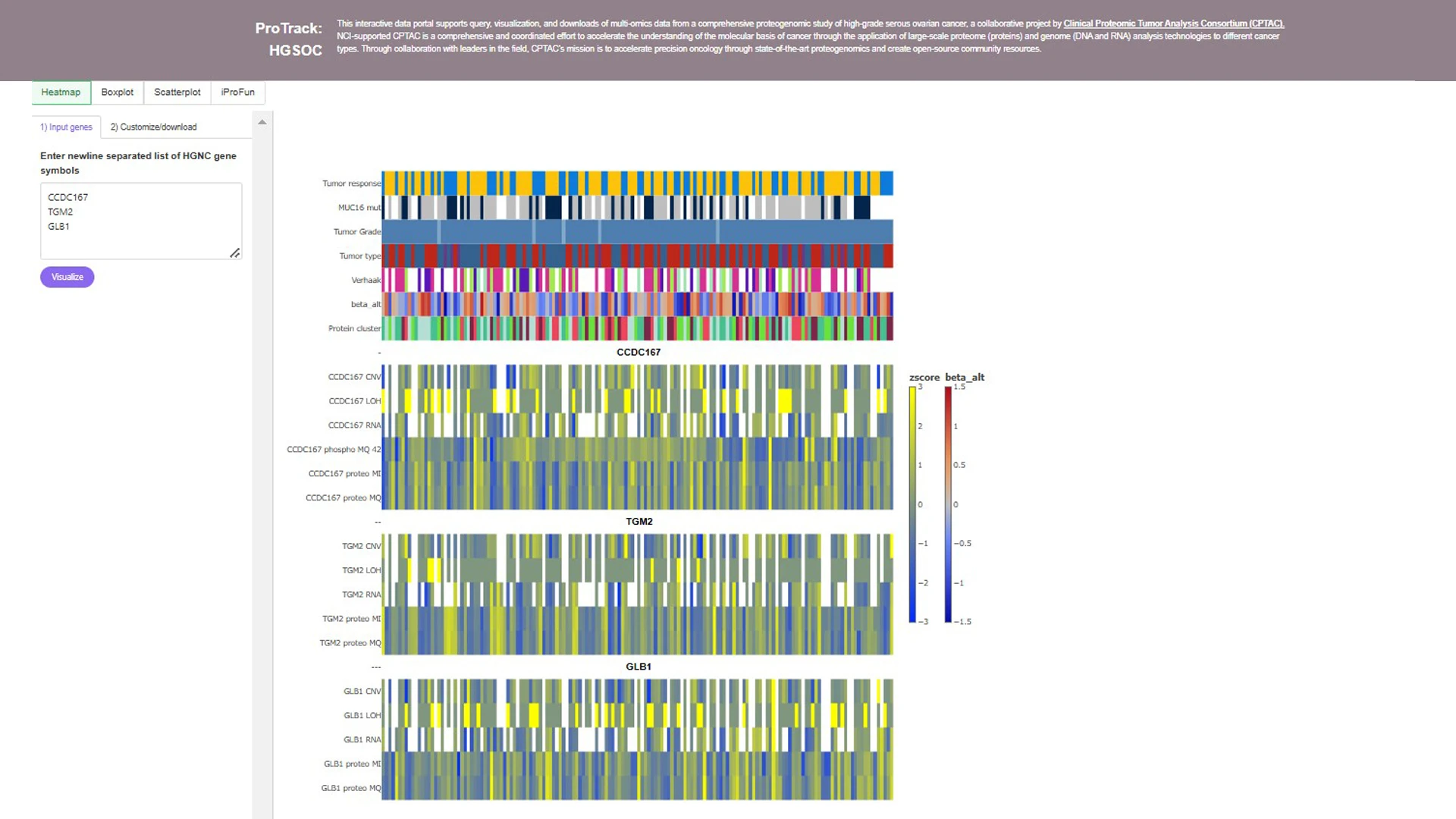
Dr. Wang has developed various bioinformatics software tools and web applications for analyzing and accessing CPTAC proteogenomic data, including ProTrack, an interactive data portal for query, visualization, and downloads of CPTAC studies. In addition to a high-grade serous ovarian cancer study, as shown, ProTrack includes findings from a pan-cancer KEA3 study, clear cell renal cell carcinoma, pediatric brain tumor, and more.
“These results are encouraging because predictors of refractory disease could enable a precision oncology approach and provide a way to select patients for clinical trials to identify and implement effective therapies,” says Dr. Wang. “Our 64-protein prediction model, for example, can detect a subset of around 35 percent refractory tumors with 98 percent specificity.”
Dr. Wang is also using machine learning and deep learning techniques in an ongoing CPTAC melanoma study to improve the prediction of response to dual-checkpoint immunotherapies based on multi-omics profiles. Previous studies in which machine learning was key included a first-of-its-kind attempt to perform a comprehensive proteogenomics analysis across traditional histological boundaries of pediatric brain tumor biology.
Digital Technologies and Mobile Health
Smartphones have enabled generation and reporting of health data in more ways than ever. Recently, Dr. Wang is collaborating with physicians at Mount Sinai to see if smartphone-enabled data collection and direct messaging might help individuals improve their heart health. A pilot study is underway where step count data—an ingrained feature on many mobile devices today—is analyzed for clues about the user’s activity level. And in cases where that appraisal appears to show a deficiency or falloff, electronic messages could be sent encouraging higher levels of daily activity or exercise to stave off heart disease.
“If we’re able to show that activity monitoring can serve as an accurate measurement of outcomes, it could have novel potential for both public health and clinical trials,” says Dr. Wang. “That type of information could give physicians and scientists valuable clues in advance about someone’s health that could be vital to the treatment they prescribe or who they enroll in their studies.”
That infrastructure for the pilot study is a continuation of digital health efforts at Mount Sinai that span nearly 10 years back. Then, Mount Sinai was a key collaborator on the project with various organizations, and developed the Asthma Health app using the Apple ResearchKit framework. Dr. Wang designed the data analysis and interpretation component of the study, digesting data from 7,593 people who enrolled in the study.
Scientists were able to correlate increased daily asthma symptoms among study participants in Washington State, for instance, during an outbreak of wildfires. From the iPhone data stream, they were also able to detect the different subtypes of asthma affected by environmental factors, and those that weren’t. In terms of clinical follow-up, the groundbreaking study allowed for the transmission of warnings about poor air quality to individuals in the affected geographic areas, along with suggestions they carry with them and be prepared to use any respiratory therapies.
Opening New Vistas Through Multimodal Data Integration
The period ahead will be the most exciting and productive yet for computational biologists and statisticians, says Dr. Wang, as new pathways open up that reveal previously unknown linkages among complex molecular systems, lifestyles, human behavior, and health.
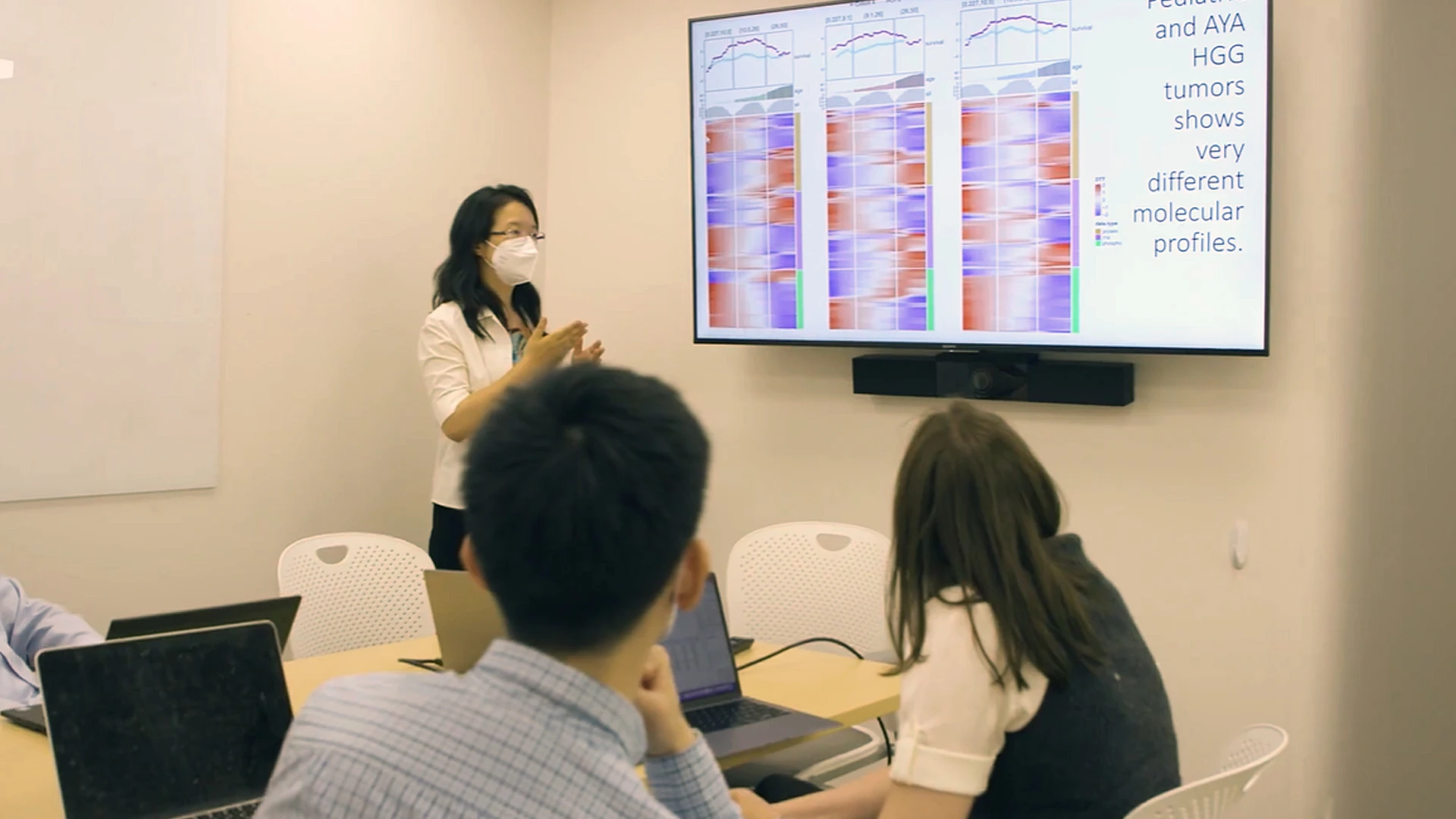
Dr. Wang is excited about the new discoveries to be made in the ever-changing multi-omics field, especially as AI and machine learning technologies and methods advance. In particular, she is looking at cross-omic comparisons and analyses, including genomics versus proteomics, and integration of the various -omic segments via big data methodology.
Dr. Wang believes she will be able to help define how the field moves forward, especially with her work with CPTAC and at Mount Sinai. She is looking at developing new methodologies for multimodality data integration that carefully weigh genomics versus proteomics, bulk versus spatial and single-cell data, and integration of -omics with electronic health record data. These eventually point to a goal of enhanced prognostics and treatment of all types of cancer, and the key to that effort will be machine learning, deep learning, and AI techniques for complex biomedical datasets.
“From data flows knowledge, and the more molecular data we have, the better our understanding of the mechanics of cancer and how we can inform patients of the most appropriate treatment strategies for their subtype of tumor,” says Dr. Wang.
Featured
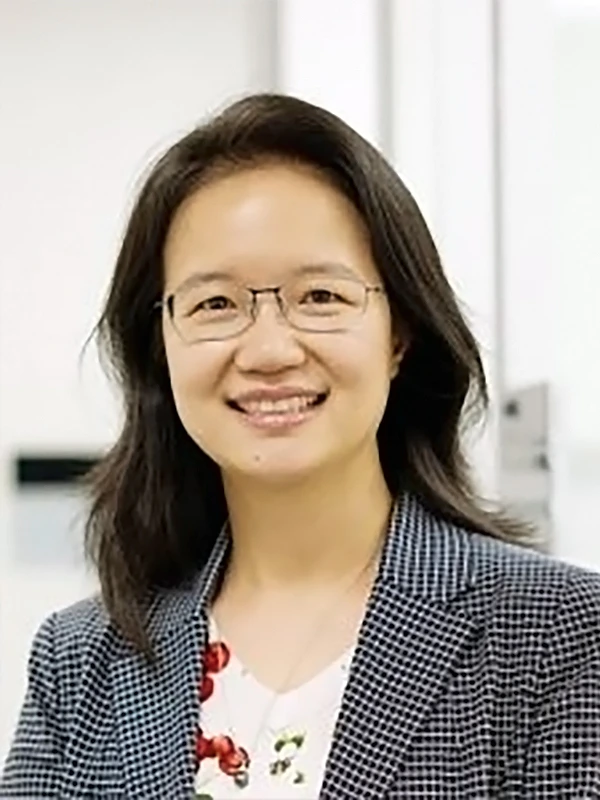
Pei Wang, PhD
Professor of Genetics and Genomic Sciences