Despite the gains sleep medicine has made in analyzing and treating obstructive sleep apnea (OSA), clinicians still rely heavily on the apnea-hypopnea index (AHI), a rather crude measure of how many times over the course of an overnight sleep study an individual stops breathing or has reduced ventilation. The limitations of AHI are many: it fails to predict who will be responsive to treatment, who will benefit from other types of treatments or adhere to them, and, finally, whether those therapies are likely to reduce cardiovascular risk.
Sleep researchers at Mount Sinai have made a major breakthrough by developing a new model that analyzes sleep study data using generative artificial intelligence (AI), an advance that provides a robust profile of patient risk for not just cardiovascular outcomes, but all-cause mortality, daytime sleepiness, and significant OSA. Known as Physiology Guided Sleep AI, the first-of-its-kind software tool is currently being licensed to sleep researchers, with plans to commercialize it once fully validated and approved by the Food and Drug Administration, so that clinicians and patients across the country can also benefit.
“We’re combining AI models for time-series data that’s routinely collected during sleep studies with clinical notes that include detailed medical history and patient reports of symptoms to give clinicians a decision-making aid to personalize treatment for sleep apnea patients,” says Ankit Parekh, PhD, Assistant Professor of Medicine (Pulmonary, Critical Care, and Sleep Medicine) at the Icahn School of Medicine at Mount Sinai, and Director of the Sleep and Circadian Analysis Group, which is developing Physiology Guided Sleep AI.
“This will give clinicians who currently rely on arbitrary rules and thresholds a validated tool to better manage the diagnosis and treatment of sleep apnea,” Dr. Parekh says. “And for patients, it will mean a more comprehensive and quicker way to evaluate their sleep disorders so they don’t have to wait six to eight months, as they typically do now, for doctors to determine and begin the most appropriate treatment.”
OSA is believed to affect 900 million people globally, and up to 54 million in the United States. Varying widely in its intensity, OSA is associated with cardiovascular and cerebrovascular disease, neurocognitive impairment, and daytime sleepiness. The Mount Sinai Sleep and Circadian Analysis Group is committed to quantifying the massive amounts of data from overnight sleep labs and mobile devices that patients bring home by applying machine learning and AI methodologies, along with mathematical modeling, to provide clinicians with state-of-the-art predictive tools.
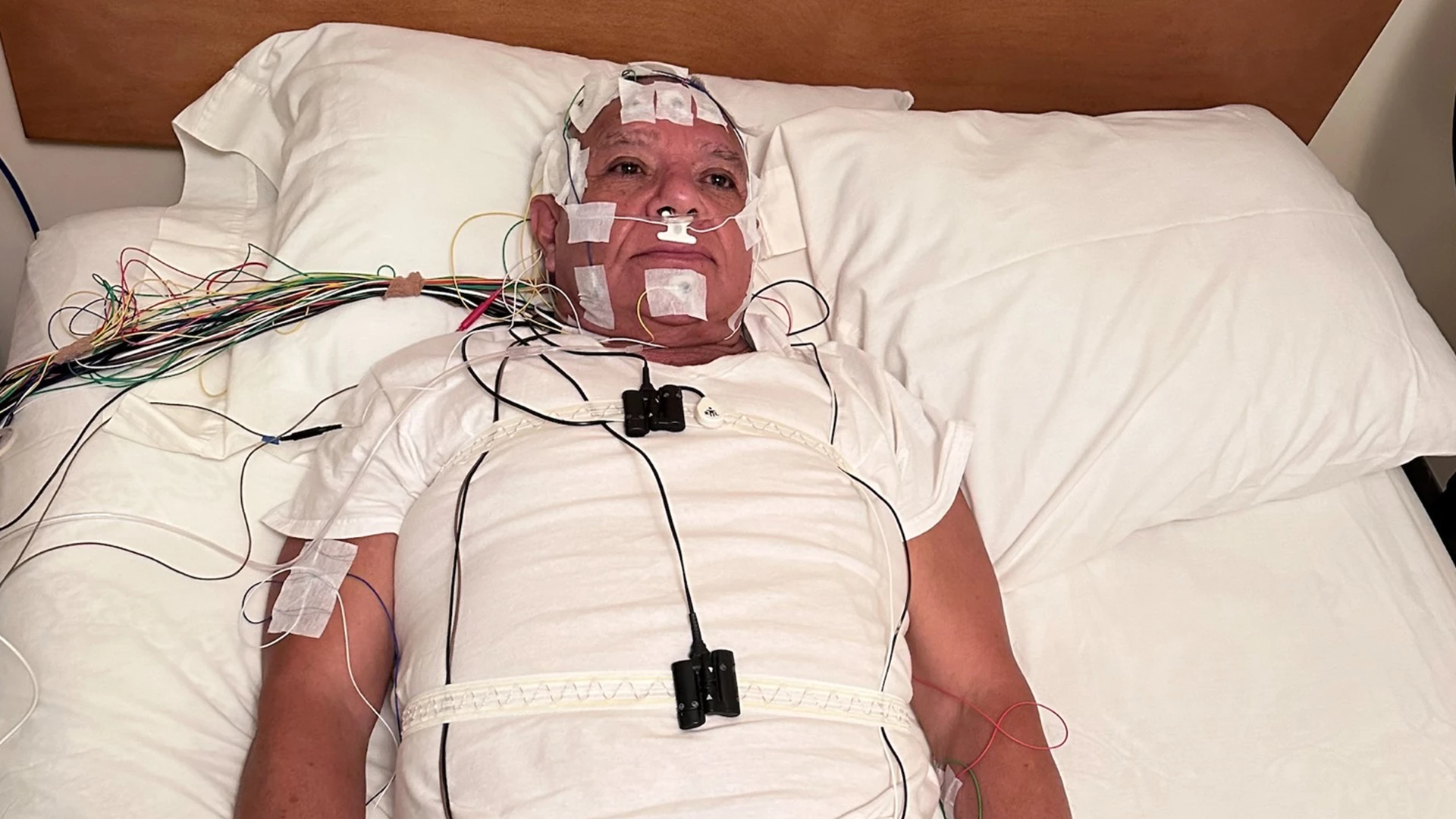
Sleep lab studies generate massive amounts of data that Mount Sinai researchers are now analyzing with artificial intelligence.
At the core of the group’s Physiology Guided Sleep AI model is an innovative framework for combining and then synthesizing polysomnography-gathered data from three different “burdens” to arrive at a risk profile for the patient, ranging from the least serious (stage one) to the most serious (stage four) across various clinical outcomes. One of these machine learning-weighted burdens is ventilatory, a breath-by-breath measure that assesses the proportion of small breaths during a routine sleep study while showing its relationship to obstruction in the upper airway. The second burden is hypoxic, which quantifies the level of oxygen desaturation overnight, and thus suggests the frequency as well as the depth and duration of sleep-related upper airway obstruction. The final burden is arousal intensity derived from EEG, to ascertain the time spent awake overnight.
“Instead of one metric that fits all obstructive sleep apnea outcomes, we’ve trained our algorithm using more than 30,000 sleep studies from a diverse group of sleep apnea patients to recognize and assess the probability of a patient having one or multiple outcomes, including daytime sleepiness, cardiovascular irregularities, all-cause mortality, and significant OSA,” explains Dr. Parekh, who holds advanced degrees in applied mathematics and computer engineering. “Depending on the type and number of outcomes, the patient could be classified as high or higher risk, which would greatly affect how they are treated.”
Among the more recent treatments for sleep apnea that clinicians might consider are hypoglossal nerve stimulation, which uses an implant to stimulate a nerve under the tongue to prevent it from blocking the airway, or oral appliances placed in the mouth to keep the patient’s airway open during sleep. Currently, it’s not feasible to determine the best candidates for these treatments versus standard sleep apnea therapies based on their sleep studies alone, a shortcoming the new AI-driven model attempts to solve.
The Physiology Guided Sleep AI model will undoubtedly find a highly receptive audience among sleep disorder specialists. “They currently synthesize in their heads a lot of the information they read from sleep studies, looking for things such as changes in breathing and oxygen desaturation,” says Dr. Parekh. “Our AI-based tool would give them, for the first time, an evidence-based and quantifiable tool for evaluating those parameters and then making the best treatment decisions possible on behalf of their patients with obstructive sleep apnea.”