Artificial intelligence (AI) is starting to transform sleep medicine, applying its ability to make sense of large datasets to the massive amounts of data generated from overnight sleep laboratories and, more recently, mobile devices that patients bring home to monitor and record their sleep-related breathing activity.
The Mount Sinai Health System, through its clinical and research programs for sleep disorders, is a leader in the science of developing automated approaches based on advanced pattern recognition. This approach has the potential to improve diagnosis and treatment of sleep disorders such as obstructive sleep apnea—which affects more than 1 billion people worldwide—while providing new insights into the mechanisms of sleep pathology at both patient and population levels.
In recent years, AI and machine learning have made inroads in the field through commercially available Food and Drug Administration-approved software programs whose algorithms help technologists at sleep labs score or interpret data. This pertains particularly to data gathered by mobile sleep devices, and infrequently to polysomnography (PSG) data, which are still largely scored manually by sleep technicians in major academic centers. Among the data collected are brain waves, oxygen levels, heart rate, breathing, and eye and limb movements.
But even that broad array of data yields limited results, as sleep medicine therapists await the development of more sophisticated algorithms that can identify polysomnogram features that often go unrecognized today by the human eye. As a result, reams of potentially helpful sleep data are left on the table.
“Polysomnography records roughly 15 different channels of data, yet we currently use only three or four of them in assessing obstructive sleep apnea,” explains Neomi Shah, MD, MPH, Professor of Medicine (Pulmonary, Critical Care, and Sleep Medicine) at the Icahn School of Medicine at Mount Sinai, who is both a sleep researcher and clinician. “That is why AI is so ripe for our field: because it could allow us to leverage all of the data in a comprehensive way to better inform clinical decision-making and improve patient outcomes.”
Mount Sinai’s Sleep and Circadian Analysis Group is working tirelessly to marry AI and sleep medicine. At a time when physicians and sleep technicians visually examine data from an eight-hour sleep study to identify abnormal patterns that appear to represent disease states, the Group employs extensive accumulated data and experience to develop AI-based predictive models that can not only automate the scoring of sleep-related events, but also evaluate risk of adverse outcomes for patients with sleep-disordered breathing. This work includes developing automated approaches that quantify abnormal patterns in sleep electroencephalograms (EEGs) and enable better definition of disease burden in sleep apnea, and making these findings publicly available.
“We’re now able to analyze data in ways and at speeds we never could before, which allows us to put this processing power and expertise in the hands of researchers around the world,” says Ankit Parekh, PhD, Assistant Professor of Medicine (Pulmonary, Critical Care, and Sleep Medicine) and Director of the Sleep and Circadian Analysis Group. “The scientific community is increasingly focused on understanding the various endotypes and phenotypes of obstructive sleep apnea as a pathway to tailoring treatment to each patient.”
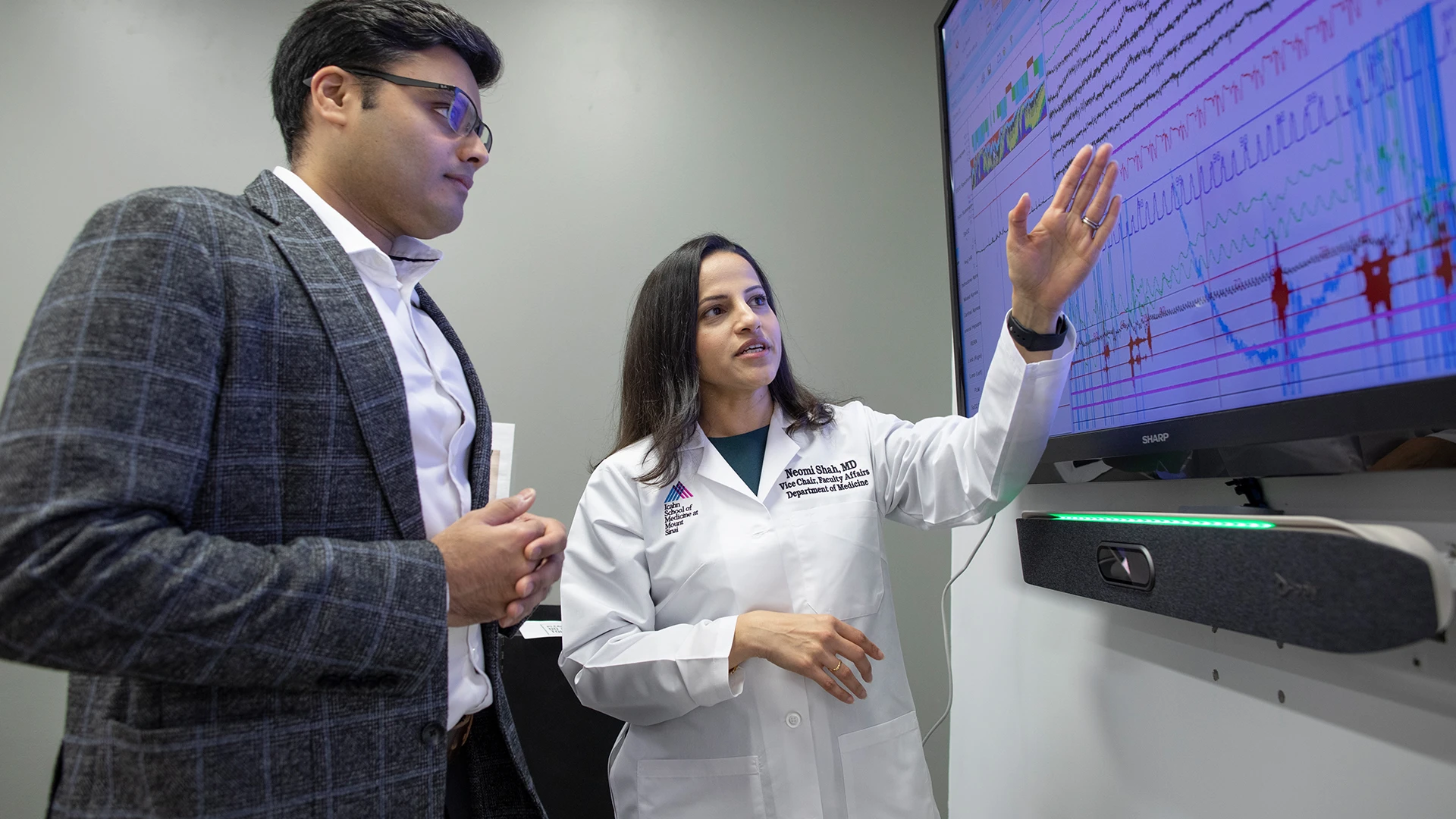
Ongoing work by the Group is aimed at putting these validated tools in the hands of clinicians and researchers at the University of California San Diego, Stanford University, Harvard Medical School, and more than 30 other institutions globally.
Dr. Parekh is also focused on revolutionizing the technology for sleep treatment clinics. To that end, his team has made considerable progress developing a machine learning application—packaged as easy-to-use software—that would deliver a robust set of metrics using multimodal data. This application would allow clinicians and technicians to draw insights from PSG that go well beyond the routinely used summary metrics and indices that often fail to correspond with meaningful clinical outcomes for patients with sleep disorders.
As the American Academy of Sleep Medicine pointed out in a recent study, AI applied to the new generation of mobile sleep testing devices brought home by users could identify positive airway pressure (PAP) adherence and mask-fitting difficulties, allowing for patient fixes that could increase adherence to treatment. Just as important, because PAP users often have comorbidities such as heart disease, newly revealed signatures in the data could provide a window into heart failure or other debilitating diseases downstream—hopefully in time to initiate preventive measures. Moreover, AI technology could help distill patient data to pinpoint which treatments are working or, conversely, why they’re not.
“AI is clearly the direction in which we want to take the field of sleep medicine, even if we’re not there yet,” observes Dr. Shah, who is working closely with Dr. Parekh on the new sleep medicine data processing application. “Once we can predict outcomes from the data we’ve amassed, we’ll be able to identify and prioritize sub-groups that will benefit most from specific treatments, as well as take steps to ensure their adherence. If we know, for example, that a patient’s risk of heart attack is 10 times that of someone else with obstructive sleep apnea, we could channel more resources into ensuring they’re using their PAP for eight hours instead of just four.”