Related Article
A simple tool that accurately calculates a patient’s readiness to be liberated from a mechanical ventilator has long been a holy grail for intensive care unit (ICU) clinicians. Pranai Tandon, MD, believes he may have found it.
Dr. Tandon is assessing the potential of applying artificial intelligence to clinical data to enable more accurate assessments of patient readiness. Working with Icahn School of Medicine at Mount Sinai colleagues in Pulmonology, Clinical Data Science, and Data-Driven and Digital Medicine, he has developed and is studying a predictive algorithm that uses a range of electronic medical record (EMR) data such as patient breath sounds and PaO2/FiO2 ratio to identify patients who are ready to be liberated from ventilation. If successful, Dr. Tandon believes that the algorithm could be impactful for both ICUs and patients.
“Patients who are left on a ventilator too long not only experience a wide variety of health problems but also contribute to a decrease in overall ICU throughput, which has ramifications for outcomes among other patients requiring ventilation,” says Dr. Tandon, Assistant Professor of Medicine (Pulmonary, Critical Care and Sleep Medicine) at Icahn Mount Sinai.
“Liberating patients too early from a ventilator can also cause harm, whether that is from the stress of not being able to breathe easily or the need to reintubate. For these reasons, there is a strong desire among physicians for a simple tool or calculation to augment their expertise in making these determinations. Machine-learning algorithms are a potential solution, as they are not only able to synthesize the wide range of data necessary to make these complex decisions but also can provide customized predictions for each patient.”
Liberating a patient from ventilation neither too early nor too late
The inspiration for Dr. Tandon’s algorithm originated with ReSCUE-ME (Realtime Streaming Clinical Use Engine for Medical Escalation), an innovative Mount Sinai clinical trial that assessed the potential to use machine-learning algorithms to predict clinical deterioration. A literature review revealed several successful studies involving predictive algorithms, but Dr. Tandon notes that they were typically small, and the algorithms could not be applied in patient care. “We have a large patient cohort, we are streaming data from patients, and we have a clinically focused team. For those reasons, I saw that we could potentially develop an algorithm that we could weave into actual care,” Dr. Tandon says.
Initially, Dr. Tandon developed a simple algorithm to collect data on intubation and extubation times from the EMRs of patients in The Mount Sinai Hospital ICU, which are updated every two minutes. He then partnered with the department’s respiratory therapists for a two-month study to validate his findings. He demonstrated that the algorithm captured more accurate intubation and extubation times than those recorded by hand. Dr. Tandon subsequently looked at other variables, such as average number of patients intubated at any given time in the ICU, time of day that extubation typically occurs, and percentage of patients who are reintubated. These variables demonstrated an opportunity for improvement of the predictive algorithm, which Dr. Tandon trained on a cohort of 2,615 Mount Sinai patients who underwent an extubation attempt at least once between 2011 and 2019. The cutoff was chosen to exclude COVID-19 patients, due in part to low rates of successful ventilator liberation, and to evolutions in best practices and patient populations that would render any model obsolete.
“We split them into testing, training, and validation training sets so we could decide which variables should ultimately be included in the model,” Dr. Tandon says. “The process requires a considerable degree of experimentation in which you generate hundreds of different possible models and then pick the best-performing one for validation.”
The model that Dr. Tandon chose for validation included predictive factors such as level of consciousness, PaO2/FiO2 ratio, lactate production, and patient breath sounds. The latter, which was the most powerful predictor, are categorized as clear, rhonchi, wheeze, diminished, or null (i.e., absent data) based upon twice-daily assessments and descriptions provided by a respiratory therapist or nurse. The model produces numeric probabilities from 0 to 1, but for the purposes of validation, Dr. Tandon used a false positive/true positive balance threshold of 0.45, resulting in a positive predictive value of 89.5 percent and a negative predictive value of 50.6 percent.
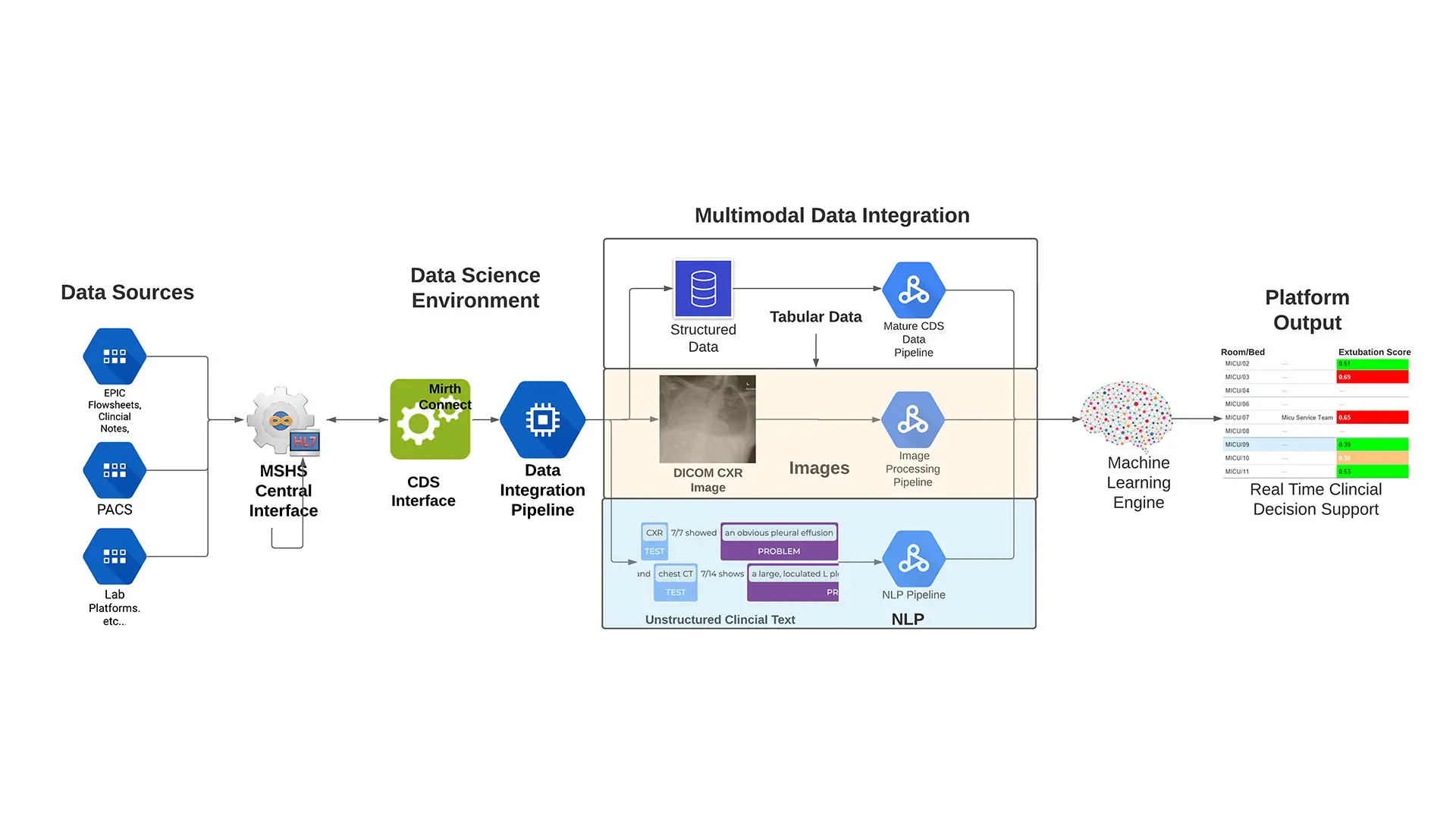
Flow chart of the algorithm
Using the historic cohort, Dr. Tandon compared the predictive potential of this model against the rapid shallow breathing index—the most used index for determining patient liberation readiness—at the traditional threshold of 105 breaths/minute/liter, which has a positive predictive value of 85.5 percent and a negative predictive value of 11.8 percent. He found that his model achieved an area under the curve of 75 percent versus 54 percent for the index. “What we observed was that the algorithm was similar to the index in terms of patients who were ready for extubation, but the difference was that the algorithm was more intelligently selecting the patients in which the decision for extubation was no,” Dr. Tandon says.
But the question remains as to how well the algorithm is able to make predictions based on live data. Dr. Tandon is currently analyzing the results of a three-month study in which the algorithm was fed live EMR data from the ICU each day at 5 am. The results, which he is reviewing with his respiratory therapy colleagues, could necessitate further tweaks to the model or a return to the drawing board. But success would enable him to proceed with a pilot study that demonstrates the potential impact of this tool.
“Our hope is that we achieve an algorithm that enables us to safely decrease the time patients spend on ventilators, enabling us to achieve improved outcomes for them,” Dr. Tandon says. “Even a decrease of 12 hours would greatly revolutionize the throughput of the ICU and enable us to achieve cost efficiencies in care.”
Featured Faculty and Division Leadership
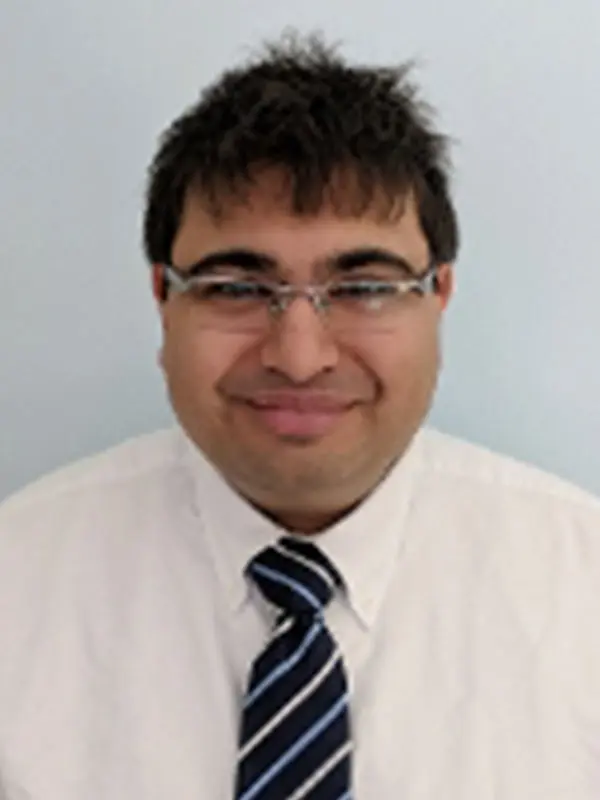
Pranai Tandon, MD
Assistant Professor of Medicine (Pulmonary, Critical Care and Sleep Medicine)
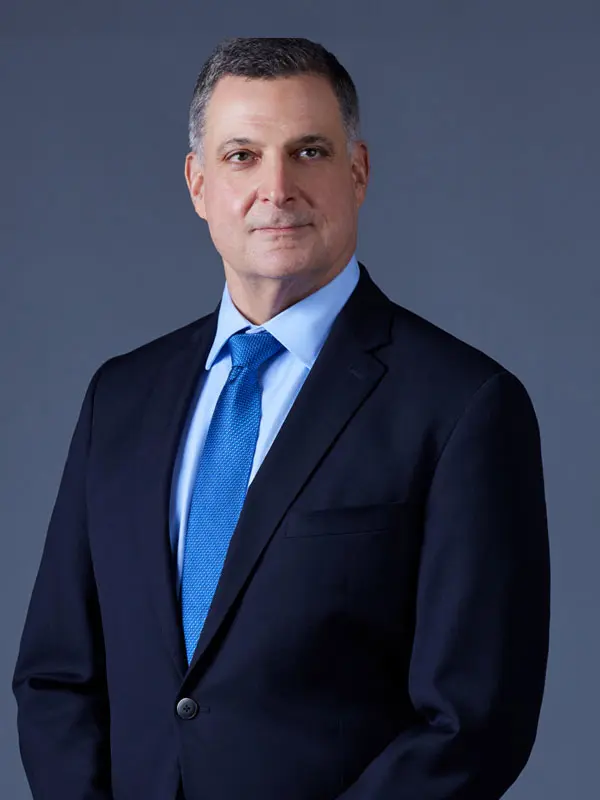
Charles A. Powell, MD, MBA
Chief of Pulmonary, Critical Care and Sleep Medicine; Chief Executive Officer, Mount Sinai – National Jewish Health Respiratory Institute