A research team of data engineers, informaticists, and physicians at Mount Sinai Health System is using artificial intelligence and machine learning to develop novel tools to interpret cardiac diagnostic studies in patients with congenital heart disease. The team is poised to transform outcomes for patients with congenital heart conditions such as tetralogy of Fallot.
Led by Sonny Duong, MD, MS, Assistant Professor of Pediatrics (Pediatric Cardiology) at the Icahn School of Medicine at Mount Sinai and the Mount Sinai Children’s Heart Center, the team is bridging the gap between modern advances in artificial intelligence and clinical medicine. They have developed a neural network-based electrocardiogram (ECG) interpretation algorithm for the analysis of patients’ ECG waveforms. This innovative tool is designed to predict the presence of right ventricular dilation and dysfunction based on cardiac magnetic resonance imaging (MRI) reference standards (see figure below).
This advancement has paved the way for novel screening techniques aimed at detecting right ventricular pathology. The researchers have also developed machine learning algorithms for prediction of cardiac MRI pulmonary regurgitation fraction, right ventricular volume, and ejection fraction from two-dimensional echocardiographic measurements.
These predictions may provide a more accurate assessment of patients with tetralogy of Fallot, the most common cyanotic congenital heart disease condition, which occurs in about 1 in every 3,000 babies born per year. Children with tetralogy of Fallot require lifelong monitoring and it is difficult to assess their hearts accurately using traditional methods. As a result, advanced cardiac imaging techniques are needed, but these can be resource-intensive and lead to delays in the delivery of care. In addition, this patient population is at risk for long-term poor health outcomes, and identifying which patients are most at risk is a clinical conundrum.
“As we expand our research, we will work on combining models to make predictions using multimodality diagnostics. We are also building multicenter collaborations to test and validate these models, as well as examine important clinical outcomes which cannot be examined at the single-center level,“ says Dr. Duong, who is also a member of the Mindich Child Health and Development Institute at Icahn Mount Sinai and of the Augmented Intelligence in Medicine and Science Laboratory in The Charles Bronfman Institute for Personalized Medicine. “These tools allow us to extract more information from traditional diagnostics using the power of big data, thus allowing us to develop the precision medicine tools needed to care for these vulnerable populations.”
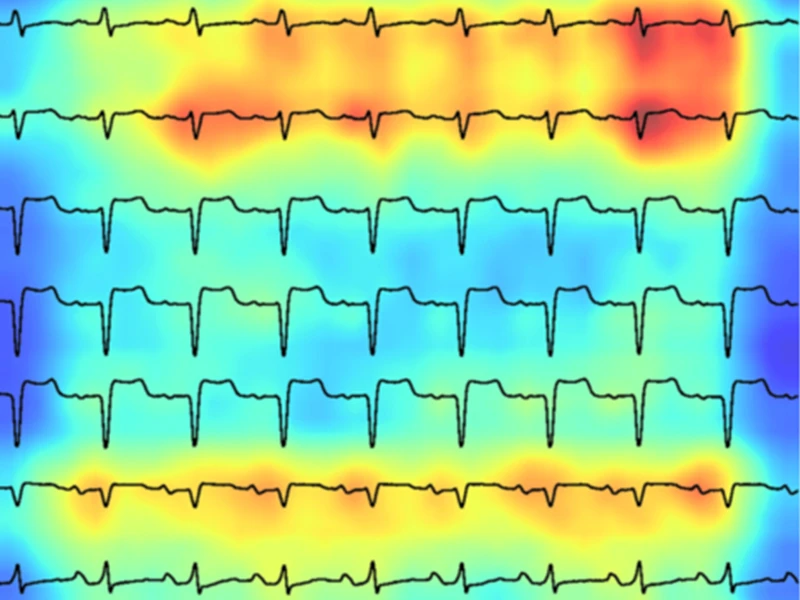
A deep learning-based ECG analysis tool is able to identify patients at high risk for poor right ventricular ejection fraction. Areas deemed important for prediction are highlighted in increasing shades of red (Duong et al, JAHA 2023).
Featured
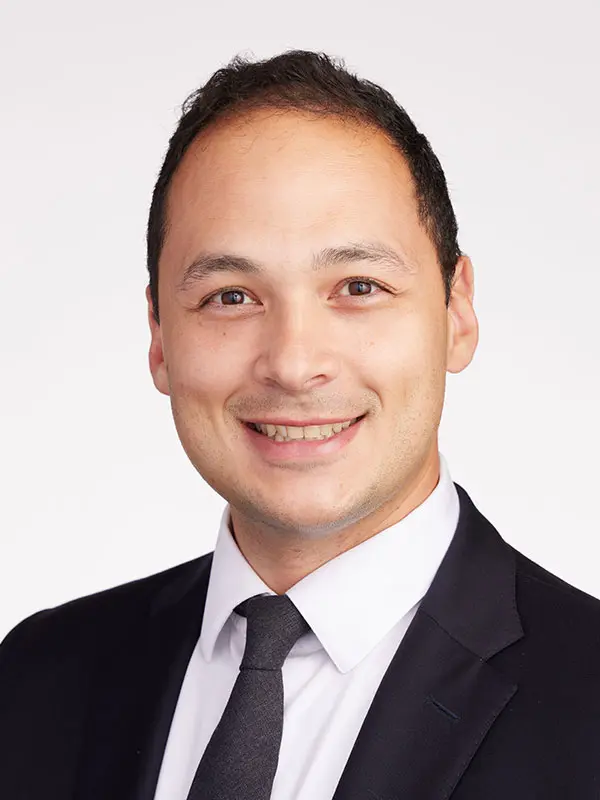
Sonny Duong, MD, MS
Assistant Professor of Pediatrics (Pediatric Cardiology)