Mount Sinai researchers in the Department of Orthopedic Surgery are working to ensure that spinal surgery is the beneficiary of breakthroughs in artificial intelligence (AI). In a study published in Spine, the team reported that machine learning, a form of AI, outperformed standard benchmark scores from the American Society for Anesthesiology (ASA) to identify risk factors potentially leading to complications following lumbar spinal fusion.
“While AI models have shown their value for analyzing and interpreting large-and-complex data sets in areas like breast and lung cancer imaging, they have yet to be used for risk-factor analysis in orthopedic surgery,” points out Samuel Cho, MD, Chief of Spine Surgery at Mount Sinai West, Professor of Orthopedic Surgery and Neurosurgery at the Icahn School of Medicine at Mount Sinai, and senior author of the study. “We learned that artificial neural networks are valid and powerful tools for predicting complications associated with spine surgery. Those findings will hopefully pave the way for their widespread use in orthopedics and other clinical fields.”
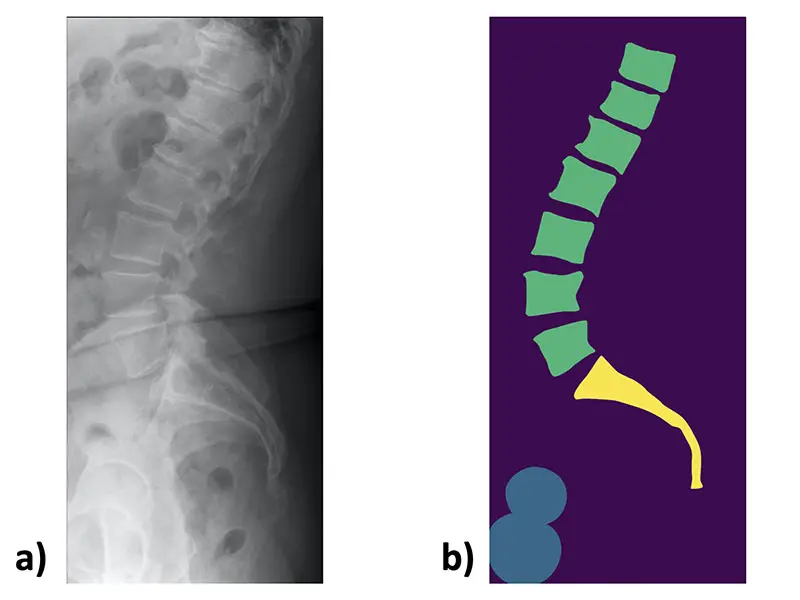
An example lateral lumbar radiograph a) and the corresponding manually created mask b)
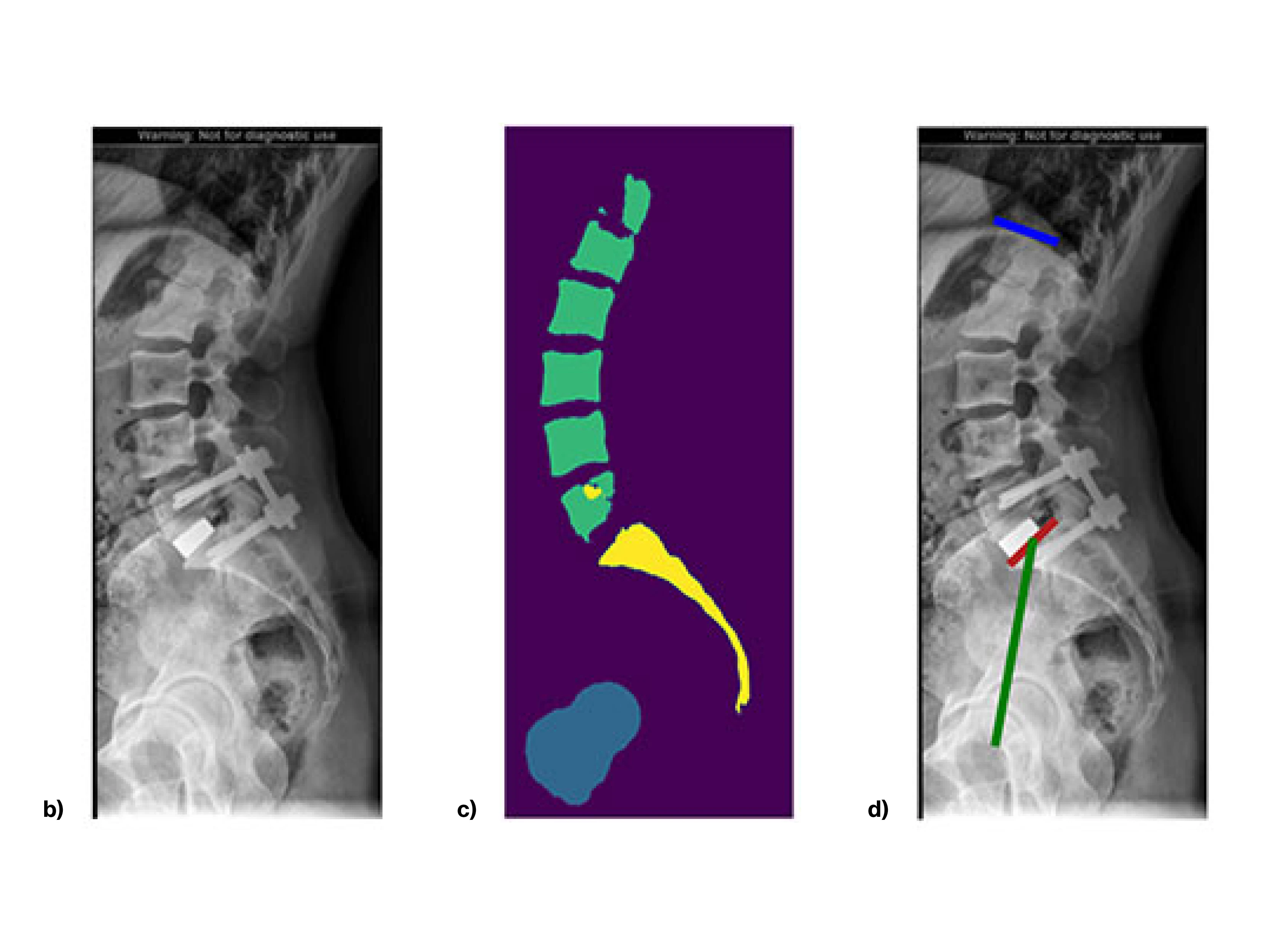
b) An input image example.
c) An algorithm-generated segmentation example.
d) An algorithm-generated measurement visualization example. Blue line indicates superior endplate of L1. Red line indicates the superior endplate of S1. Green line connects the midpoint of the S1 superior endplate with the axis of the femoral heads.
The Mount Sinai study was designed to train and validate AI algorithms to identify patient risks prior to posterior lumbar spine fusion used to treat various degenerative conditions. Algorithms like these have the ability to continuously “learn” from newly generated patient data sets and to make predictions aimed at improving the quality and efficiency of health care. For the purposes of their study, Mount Sinai researchers queried nearly 23,000 patients from the American College of Surgeons’ National Quality Improvement Program who had undergone posterior lumbar spine fusion. Among the predictive variables weighed were sex/age, ethnicity, diabetes diagnosis, tobacco use, steroid use, coagulopathy, functional status, body mass index, as well as pulmonary and cardiac comorbidities.
The investigation showed that artificial neural networks surpassed the ASA standard for predicting all four types of complications considered: cardiac, wound, venous thromboembolism, and mortality. Furthermore, artificial neural networks proved to be the most accurate for predicting cardiac complications, while logistic regression carried the advantage for wound problems.
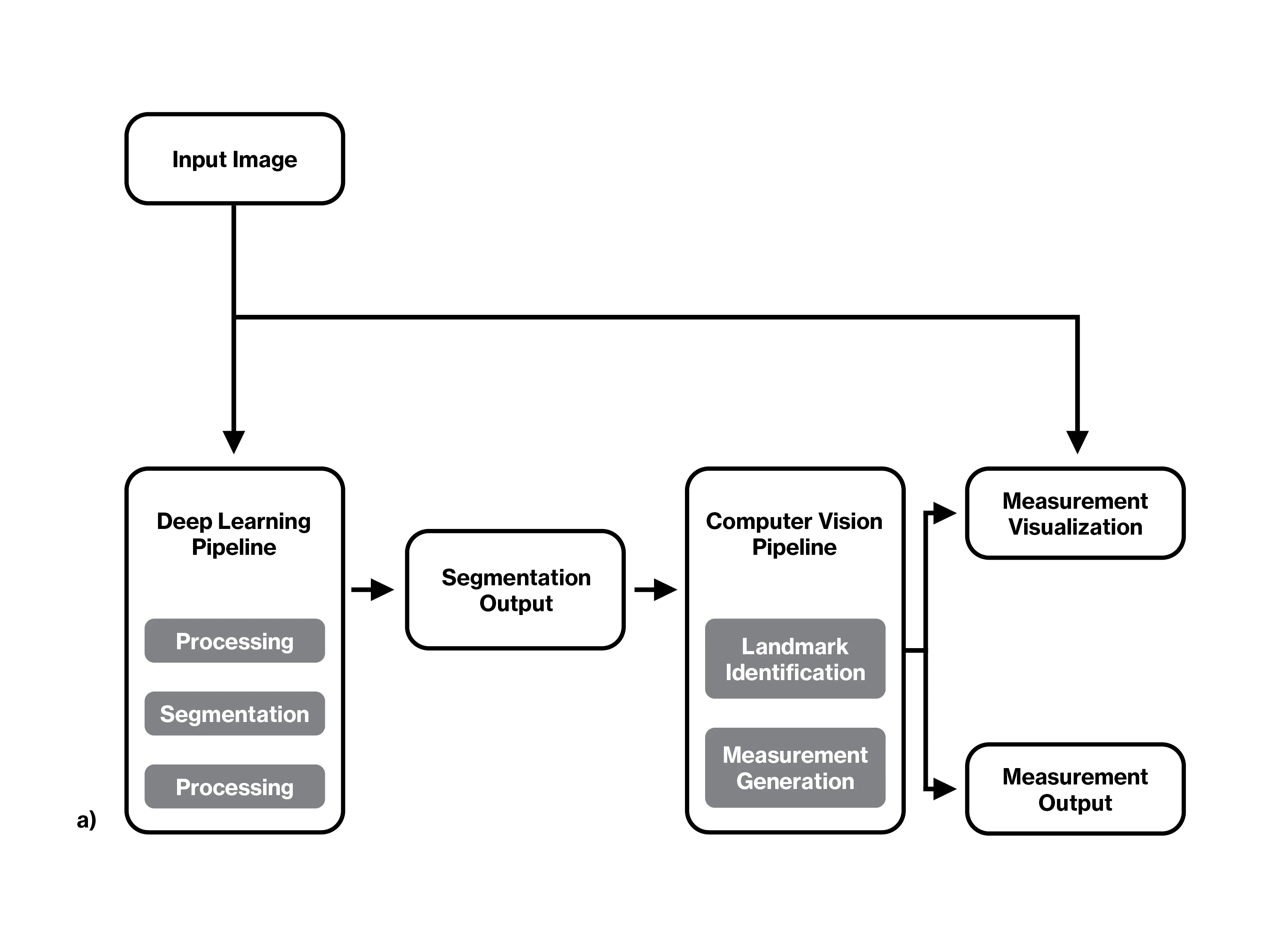
a) Flowchart of the complete algorithm pipeline.
Aside from obvious patient benefits, algorithmic surgical guidance could have a significant impact on the cost and efficiency of health care delivery. “Along with rising health care costs and greater scrutiny of surgical outcomes has come a growing emphasis on understanding risk factors in order to optimize perioperative planning and management,” explains Dr. Cho. “By reducing or preventing complications from surgery, we can clearly limit the need for additional hospitalization and other costly forms of treatment.” He elaborates that if a computer-driven analysis were to warn of the dangers from such modifiable, high-risk factors as uncontrolled diabetes, a pre-surgical patient could be referred to an endocrinologist, or in the case of obesity, to a nutritionist or gastroenterologist. The information could also figure into a discussion with the patient and family members prior to surgical intervention.
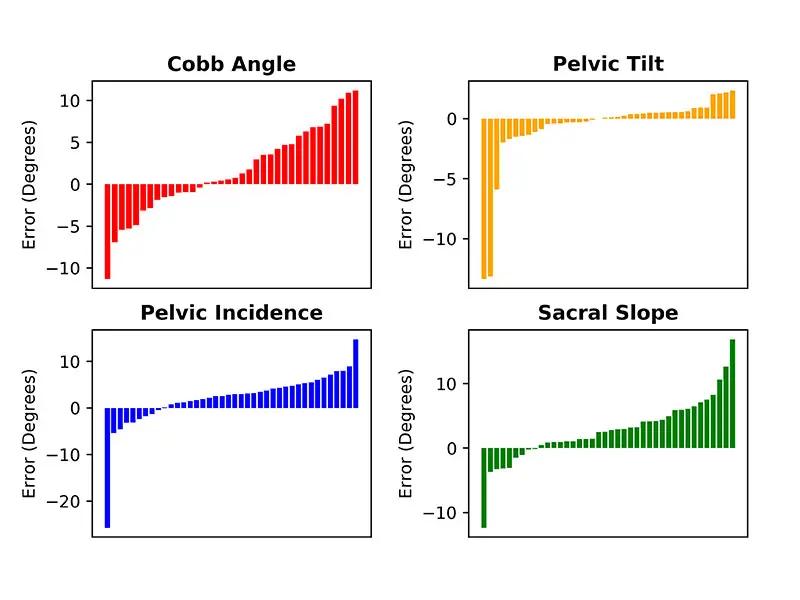
Sorted bar plots of the algorithm error for each test image measured by both the surgeons and the algorithm. Error is calculated by subtracting the average surgeon measurements from the algorithm measurements for Cobb angle, pelvic tilt, pelvic incidence, and sacral slope.
Dr. Cho sees nothing but blue sky ahead for AI—and not just in the orthopedic realm. He says, “As computing power and the ability of artificial neural networks to obtain high-quality patient data increase over time, AI techniques will become more and more commonplace in the hospital setting.”
Featured
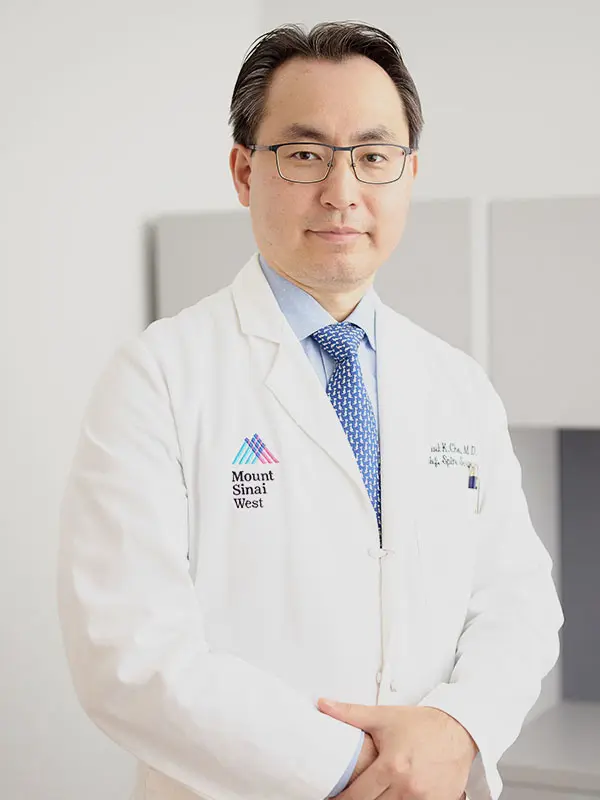
Samuel K. Cho, MD
Professor of Orthopedic Surgery and Neurosurgery