An artificial intelligence algorithm that assigns weighted grades to the traditional criteria for selecting embryos for transfer significantly improves clinical outcomes, according to a study led by Alan B. Copperman, MD, Director of Reproductive Endocrinology and Infertility, Mount Sinai Health System, and Medical Director of Reproductive Medicine Associates of New York.
“Being armed with longitudinal outcome data from thousands of embryo and patient journeys has positively impacted our ability to select optimal embryos for transfer,” Dr. Copperman says.
The investigator-initiated retrospective study was presented at the 76th Annual Scientific Virtual Congress and Expo of the American Society for Reproductive Medicine in October 2020. It included patients who underwent single-embryo transfer (SET) in the Mount Sinai Health System from September 2016 to February 2020. In one set of patients, a senior embryologist selected the embryo for transfer based on traditional modified Garner grading. In that system, the inner cell mass (ICM) and the trophectoderam (TE) cells, the outer layer that nourishes the embryo, are assigned grades depending on the number of cells present and the cohesiveness of the cell populations.
“We truly appreciate how utilization of big data assists us in improving patient outcomes.”
Alan B. Copperman, MD
In the other set of patients, selection was informed by a computer algorithm derived through machine learning, which created a weighted ranking system of each embryonic parameter—expansion, ICM grade and TE grade—and formulated a composite score. The embryo with the highest score was transferred.
Preimplantation genetic testing for aneuploidy, an abnormal number of chromosomes that can cause birth defects or miscarriage, enables modern assisted reproductive technology treatment centers to better identify and select embryos for transfer. But when patients have more than one available embryo that is euploid, containing an even number of chromosomes, a grading system provides further support in selection for transfer.
In the period studied, 4,521 SETs were performed, 1,119 using the traditional system and 3,402 using the algorithm-based system. Patients in the traditional group were older and had a lower BMI, progesterone level, and endometrial thickness at the time of transfer; demographic data were otherwise similar. The primary outcome was implantation rate (IR). Secondary outcomes were ongoing pregnancy/live birth rate, clinical loss rate, and biochemical pregnancy, in which a pregnancy is indicated by an initial test but does not progress.
The results demonstrated that using a mathematical mode for embryo selection improved clinical outcomes. The implantation rate was significantly higher in the algorithm group compared with the traditional group—63.52 percent compared with 56.93 percent. The ongoing pregnancy/live rate also was higher—53.13 percent compared with 47.18 percent. The researchers saw no significant differences in biochemical pregnancy rate and clinical loss rate.
Dr. Copperman considers the results promising. “The future is indeed bright as we begin to introduce machine-learning into the IVF laboratory,” he says. “We truly appreciate how utilization of big data assists us in improving patient outcomes.”
Featured
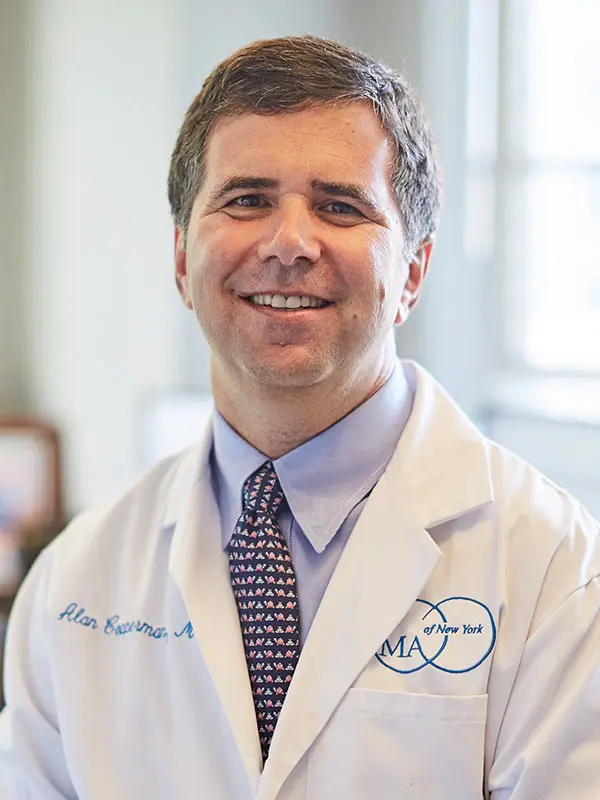
Alan B. Copperman, MD
Director of Reproductive Endocrinology and Infertility, Mount Sinai Health System, and Medical Director, Reproductive Medicine Associates of New York
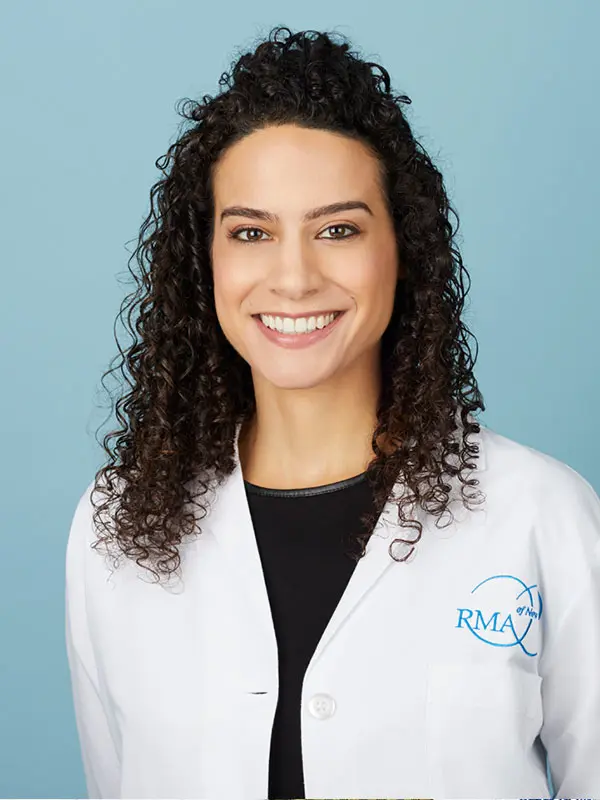
Devora A. Aharon, MD
Fellow in Reproductive Endocrinology and Infertility, Icahn School of Medicine at Mount Sinai and RMA of New York