Related Article
Data science and artificial intelligence (AI) are not only being meticulously woven into patient care across the Mount Sinai Health System, but also being deployed in innovative ways to advance both the selection and ongoing education of medical school students. One way is helping clinical fellows master the language of data analysis and science at the start of their careers; another is streamlining the medical school applications process to make it less labor-intensive for screeners and more equitable for applicants.
Mastering a New Language
The rapidly growing use of data science in everyday clinical care has underscored the need for physicians to be fluent in the often arcane medium. To that end, Lili Chan, MD, Associate Professor of Medicine (Nephrology, and Data Driven and Digital Medicine) at the Icahn School of Medicine at Mount Sinai, is developing a comprehensive Data Analysis and Data Science course.
“Much of today’s research uses machine learning, natural language processing, and other data science methods and tools, making it difficult for students who have no training in these disciplines,” says Dr. Chan, an expert in clinical research and data science. “We’re addressing this gap through a curriculum that’s focused on teaching trainees the digital skills necessary to conduct clinical research, or to simply digest the results of studies they read in leading journals that employ real-world health care data.”
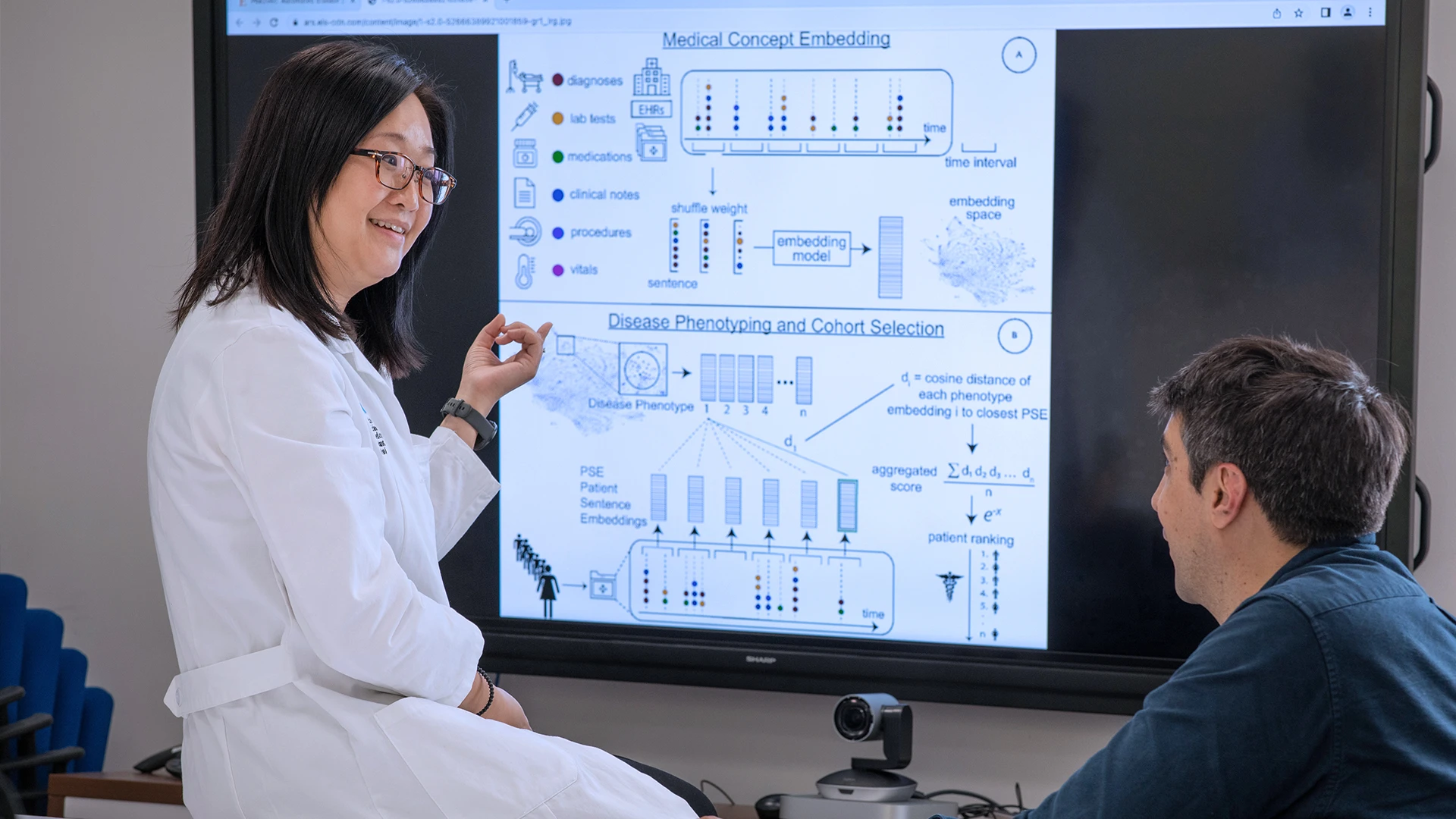
Lili Chan, MD, is developing a comprehensive Data Analysis and Data Science course.
Among topics to be covered in the lecture phase are databases, followed by the building of datasets that will be deployed through the remainder of the course. Machine learning (ML) will also be introduced, with a focus on decision trees, which form the basis for many advanced ML techniques.
“We believe this curriculum has the potential to transform trainee education,” emphasizes Dr. Chan, “by providing protected time for students to learn the data analytics and data science skills they’ll need to actively pursue research pathways.”
Streamlining Med School Admissions
No less enticing are the opportunities to leverage data science to benefit the thousands of applicants each year to Mount Sinai’s highly competitive medical school program, as well as the faculty members who voluntarily take on the task of deciding which ones get selected. Aware of the challenges, Valerie Parkas, MD, Senior Associate Dean of Admissions and Recruitment for Icahn Mount Sinai, and Talia Swartz, MD, PhD, Director of the MD-PhD program, began looking for ways that ML could help streamline the complex process.
“Every application gets read by screeners who must sort through personal statements, letters of recommendation, and related materials, which is extremely time-consuming,” explains Dr. Chan, who collaborated with Drs. Parkas and Swartz, and Justin Kauffman, a student in the Master in Data Science program, to brainstorm how ML might streamline the process. “Our goal is to reduce the number of human hours required to make sure each application is thoroughly reviewed, and to continue to ensure the process is as objective as possible.”
The team came to recognize the power and potential of a computational solution. In the same way a machine learning algorithm can be trained on reams of electronic health records to phenotype patients, so a machine learning program might be built around medical school applications to facilitate the review of candidates. “We’re still in the early data-gathering stage,” explains Dr. Chan, “but are focused on developing an algorithm through natural language processing that could identify and highlight from each application consistent themes, such as leadership or resourcefulness, which are so important within the applicant pool.”
She concludes, “We can’t remove the human element from the selection process. But we can seek to create through digital technology an equitable system for selecting students for our medical school program—one that also helps our screeners who, after all, are very busy physicians and researchers.”
Featured Faculty and Division Leadership
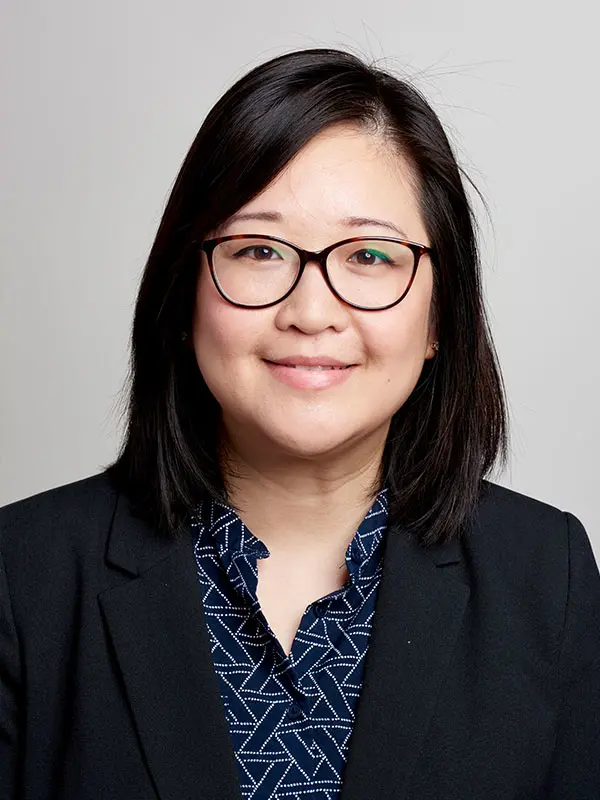
Lili Chan, MD
Professor of Medicine (Nephrology)
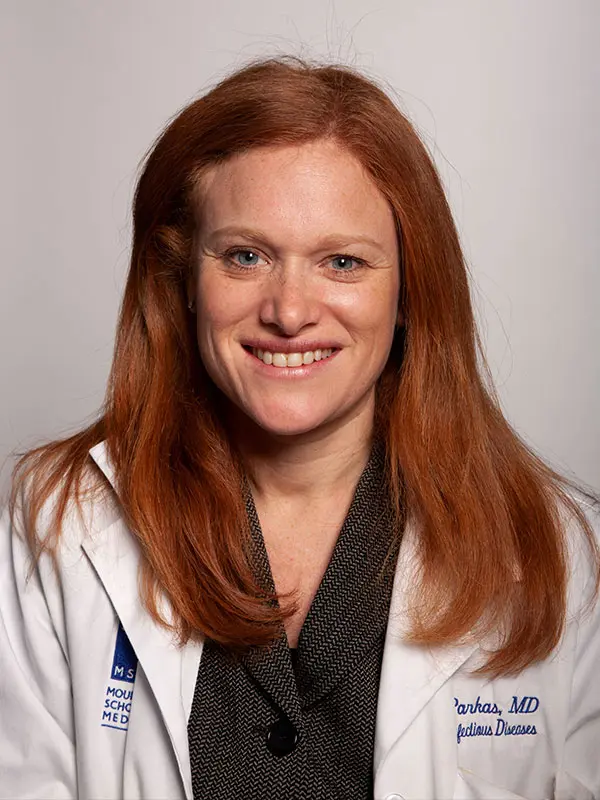
Valerie Parkas, MD
Professor of Medical Education
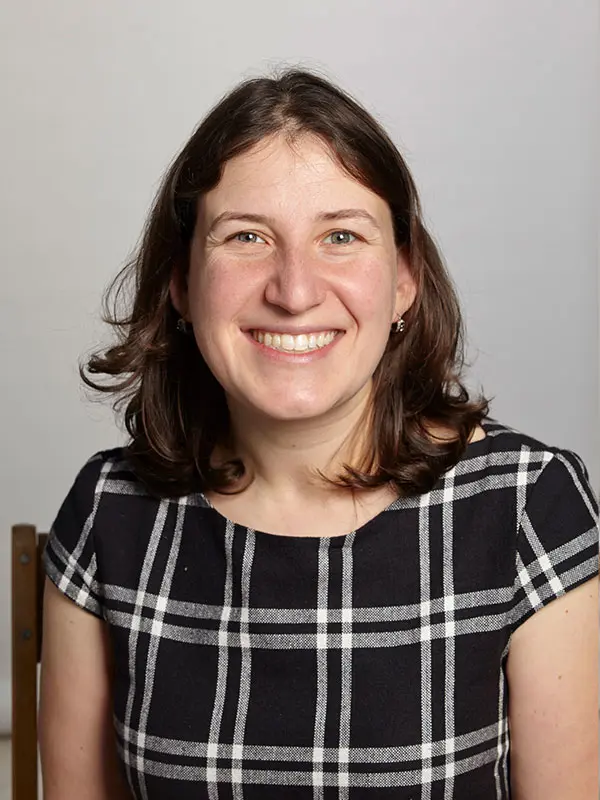
Talia Swartz, MD, PhD
Associate Professor of Medicine (Infectious Diseases), and Medical Education
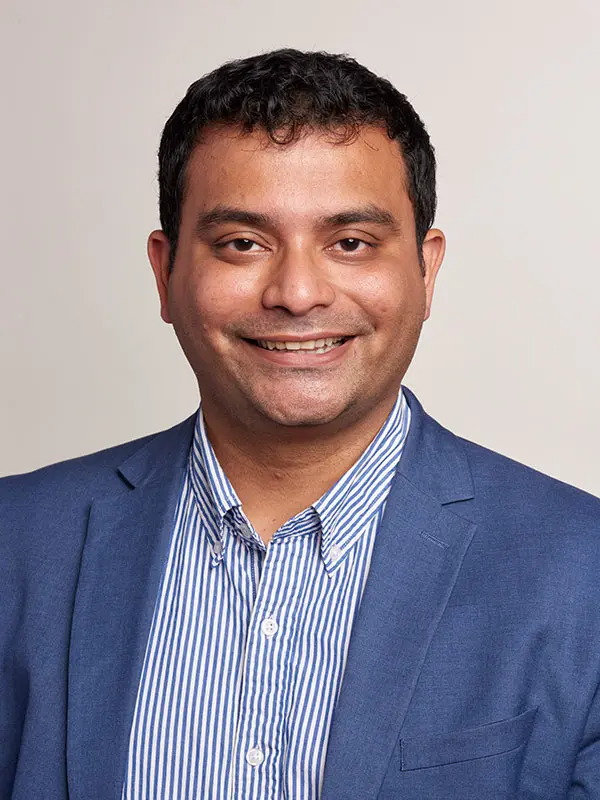
Girish Nadkarni, MD, MPH
Irene and Dr. Arthur M. Fishberg Professor of Medicine; Chief, Division of Data-Driven and Digital Medicine