Artificial intelligence (AI) has enormous potential for diagnosing and treating melanoma and other skin cancers. When it comes to diagnosis, dermoscopy, confocal microscopy, optical coherence tomography (OCT), pathology, and whole-body imaging are all areas where AI can have a great impact in improving accuracy of skin cancer diagnosis.
The Kimberly and Eric J. Waldman Department of Dermatology at the Icahn School of Medicine at Mount Sinai is working to leverage technology, specifically machine learning, to enable us to provide our patients the best care possible, both before and after diagnosis.
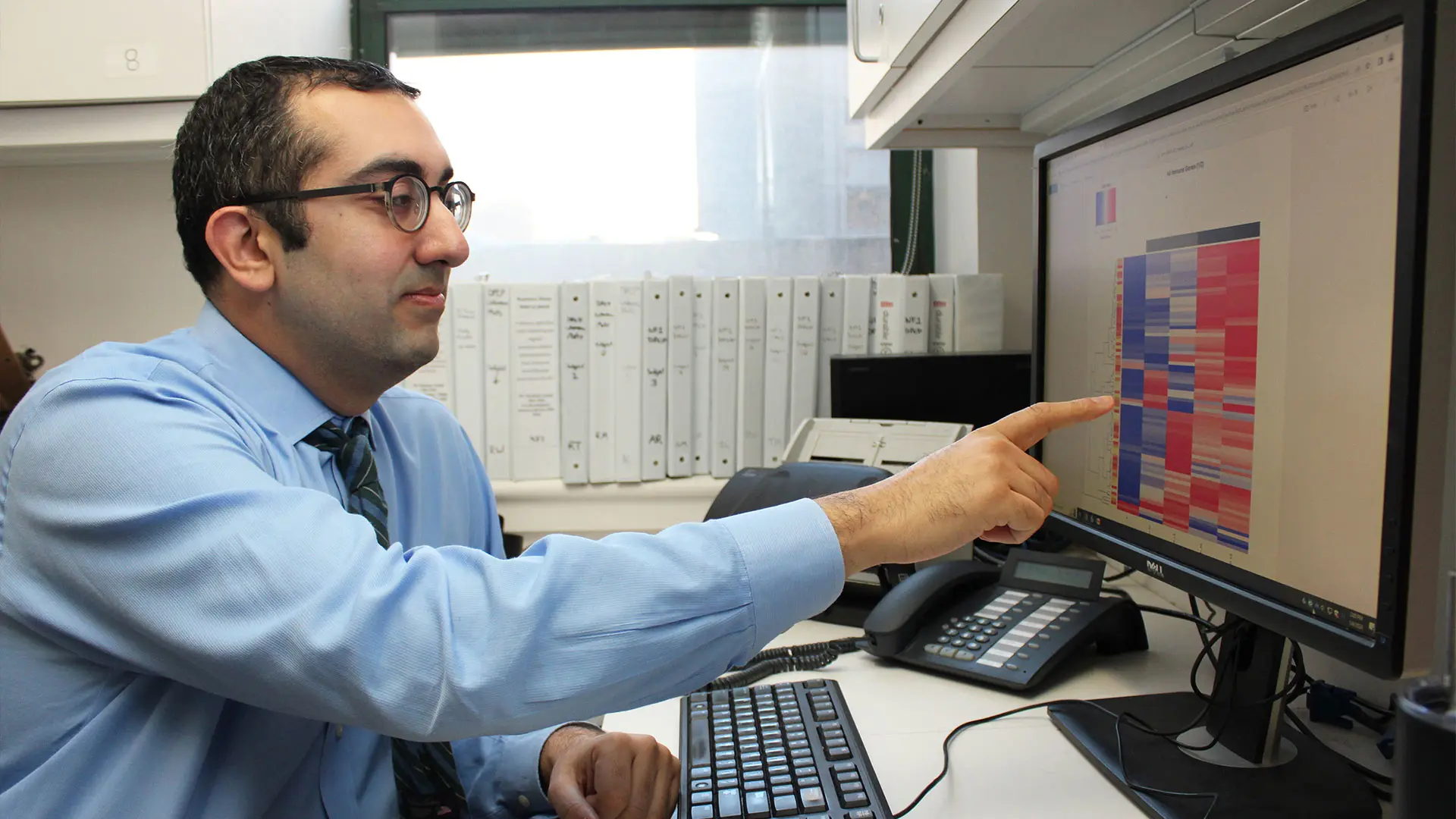
“Utilizing our various imaging technologies, and other data, we aim to develop algorithms that can not only identify individuals who are at high risk of skin cancer, but specific skin lesions that are at high risk. This would be done before a dermatologist ever looks at them, so that we can focus our attention on those lesions that pose the greatest threat,” says Jonathan Ungar, MD, Assistant Professor, Dermatology, and Medical Director of the Waldman Melanoma and Skin Cancer Center. AI has the potential to create models that will assist clinicians with the classification of these lesions into high- vs. low-risk categories.
The biggest challenge when creating AI models is having enough data to create a high-quality model. “We are currently working on collecting enough data to create such models. As an example, the Vectra, our whole-body imaging device, can potentially be used to detect high-risk lesions by comparing sets of images taken at different times, to give us information on what lesions are evolving in a concerning way and indicating which lesions have a higher chance of having malignant vs. nonmalignant potential,” says Jonas Adalsteinsson, MD, PhD, Assistant Professor, Dermatology, and Director of Dermato- epidemiology and of the Autoimmune Blistering Diseases Clinic.
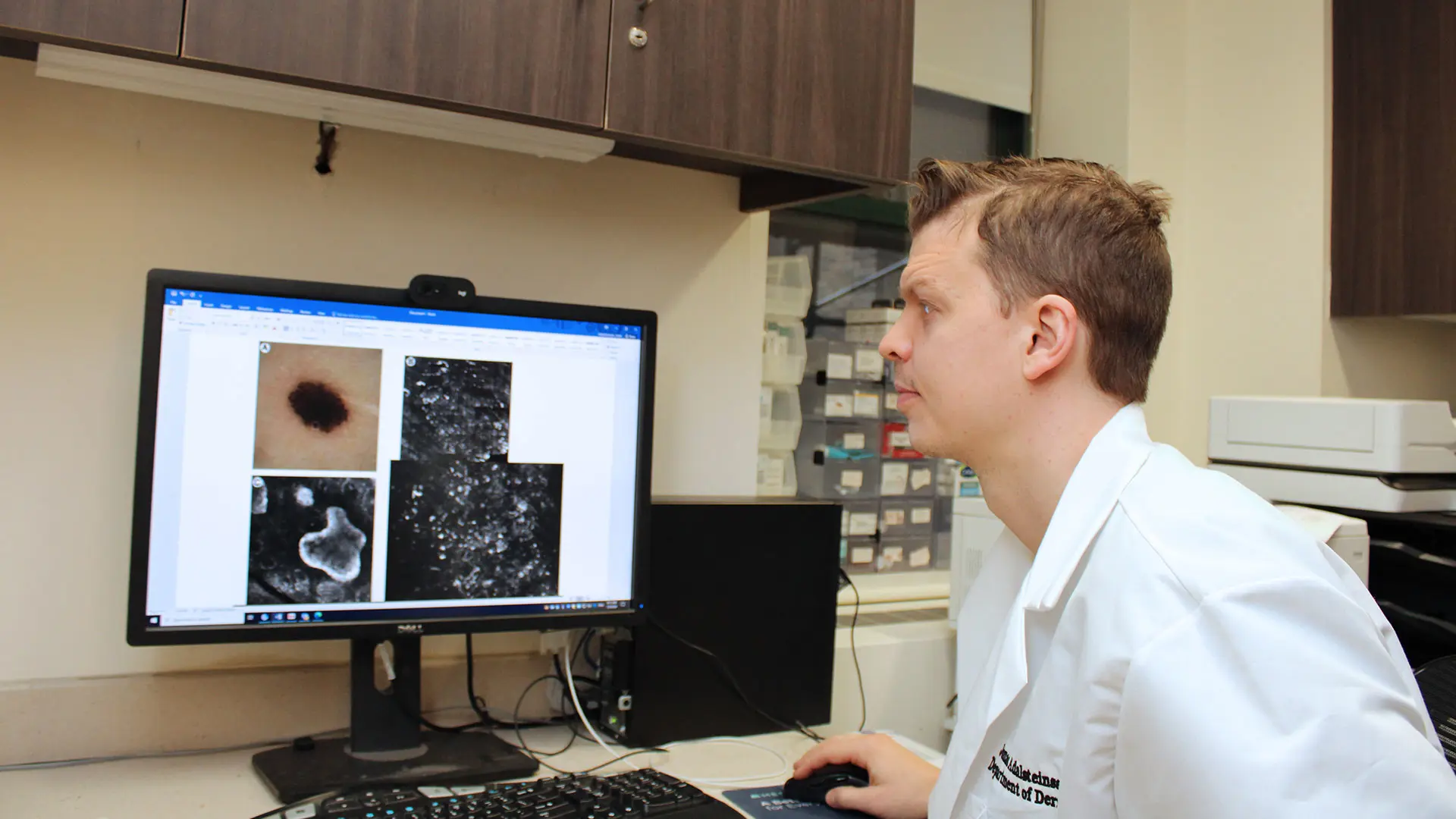
When putting patients on cancer therapy, doctors currently have no reliable way to tell which ones will have their cancer respond, and which ones will not. They also do not know which patients will develop side effects from the therapy, and these side effects can be life-threatening, or force the patient to stop their cancer therapy altogether.
“We are generating data on the expression of thousands of genes and proteins in the skin of cancer patients before and while they undergo therapy, using noninvasive tape strips that only remove the outer layers of the skin and do not leave a scar. With the assistance of AI, we hope to identify a gene or protein biomarker (or signature) that can tell us which treatment to use for which patient,” says Nicholas Gulati, MD, PhD, Assistant Professor, Dermatology, and Director of the Early Detection of Skin Cancer Clinic and the Oncodermatology Clinic
As a major clinical trials and translational research center in inflammatory skin diseases, the Department is uniquely positioned to investigate AI’s capabilities in predicting treatment responses from gene expression captured through skin biopsies as well as minimally invasive sampling methods, such as tape strips and blood draws. Our researchers have already published several papers demonstrating the ability of single and combined biomarkers to discriminate between healthy skin, atopic dermatitis, and psoriasis in both children and adults, with preliminary explorations of cutaneous single- and two-gene classifiers in predicting clinical responses to systemically administered drugs.
With increasing incorporation of tissue sampling into clinical trial protocols for diseases such as alopecia areata, hidradenitis suppurativa, and atopic dermatitis, the Department is continuing to build upon our database of clinical and transcriptomic/proteomic data collected at baseline and over time with treatment. We anticipate utilizing sequencing data and the processing power of AI to elucidate biomarkers predictive of treatment response.
We hope to use gene expression and other biomarker data to help tailor treatment approaches. Feeding this data into an appropriately trained AI would help guide the selection of better treatments targeted to specific patient needs and improve patient outcomes.